Scientific Publications
Explore our Scientific Publications
Coinvolgimento in lavori scientifici di ricerca focalizzati sull’analisi dei dati clinici.
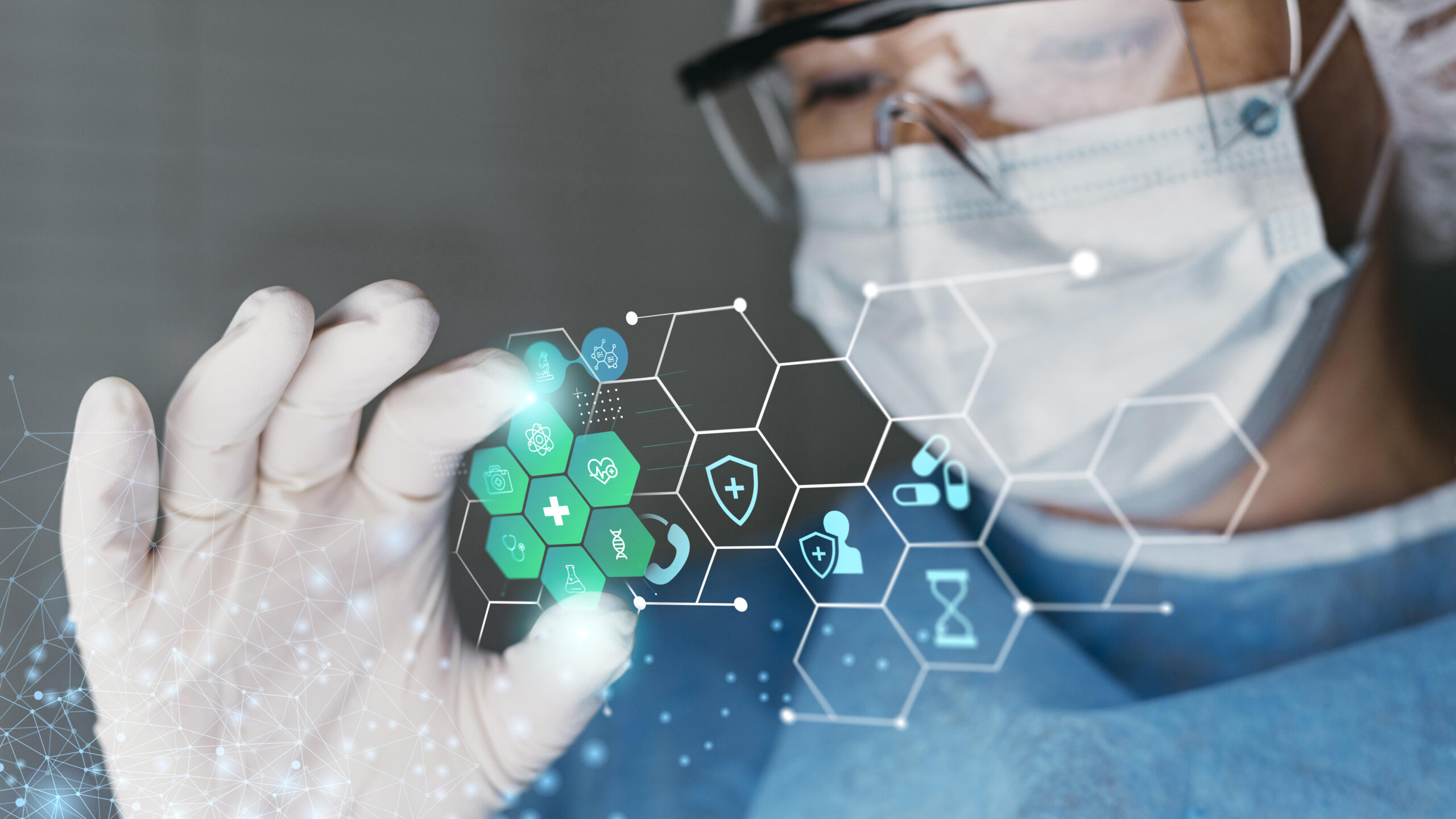
Low-pass whole genome sequencing of circulating tumor cells to evaluate chromosomal instability in triple-negative breast cancer.
Sequenziamento a bassa copertura delle cellule tumorali circolanti per valutare l’instabilità cromosomica nel tumore mammario triplo negativo.
Published: 20 SEPTEMBER 2024
Di Cosimo, S., Silvestri, M., De Marco, C. et al. Low-pass whole genome sequencing of circulating tumor cells to evaluate chromosomal instability in triple-negative breast cancer. Sci Rep 14, 20479 (2024). https://doi.org/10.1038/s41598-024-71378-3
ABSTRACT
Chromosomal Instability (CIN) is a common and evolving feature in breast cancer. Large-scale Transitions (LSTs), defined as chromosomal breakages leading to gains or losses of at least 10 Mb, have recently emerged as a metric of CIN due to their standardized definition across platforms. Herein, we report the feasibility of using low-pass Whole Genome Sequencing to assess LSTs, copy number alterations (CNAs) and their relationship in individual circulating tumor cells (CTCs) of triple-negative breast cancer (TNBC) patients. Initial assessment of LSTs in breast cancer cell lines consistently showed wide-ranging values (median 22, range 4–33, mean 21), indicating heterogeneous CIN. Subsequent analysis of CTCs revealed LST values (median 3, range 0–18, mean 5), particularly low during treatment, suggesting temporal changes in CIN levels. CNAs averaged 30 (range 5–49), with loss being predominant. As expected, CTCs with higher LSTs values exhibited increased CNAs. A CNA-based classifier of individual patient-derived CTCs, developed using machine learning, identified genes associated with both DNA proliferation and repair, such as RB1, MYC, and EXO1, as significant predictors of CIN. The model demonstrated a high predictive accuracy with an Area Under the Curve (AUC) of 0.89. Overall, these findings suggest that sequencing CTCs holds the potential to facilitate CIN evaluation and provide insights into its dynamic nature over time, with potential implications for monitoring TNBC progression through iterative assessments.
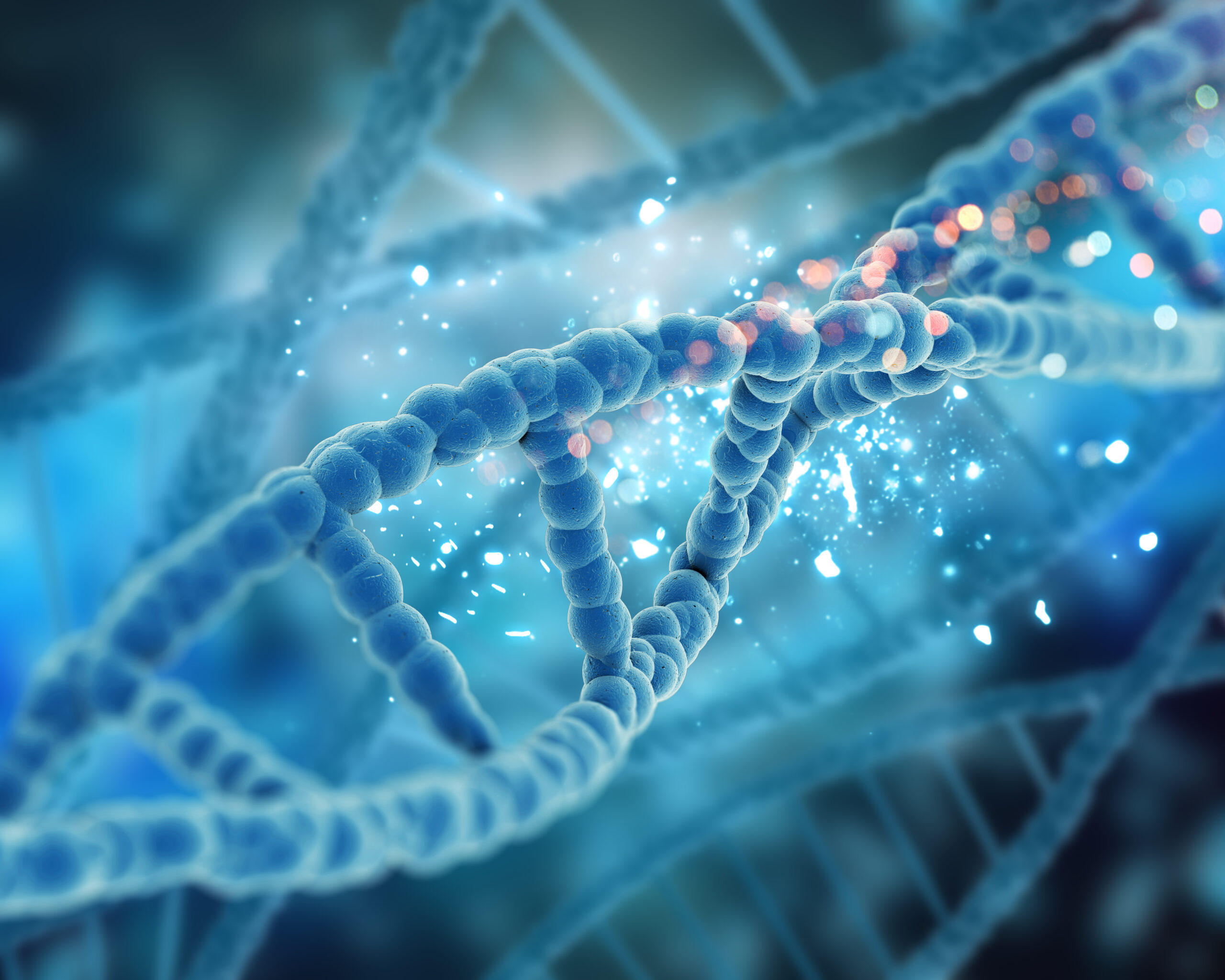
Detecting gene copy number alterations by Oncomine Comprehensive genomic profiling in a comparative study on FFPE tumor samples.
Detection of gene copy number alterations using the Oncomine genomic panel in a comparative study of FFPE tumor samples.
Published: 05 FEBRUARY 2025
Bozzi, F., Conca, E., Silvestri, M. et al. Detecting gene copy number alterations by Oncomine Comprehensive genomic profiling in a comparative study on FFPE tumor samples. Sci Rep 15, 4314 (2025). https://doi.org/10.1038/s41598-025-88494-3
ABSTRACT
Copy number alterations (CNAs) play a fundamental role in cancer development and constitute a potential tool for tailored treatments. The CNAs recognition in formalin fixed paraffin embedded (FFPE) material for diagnostic purposes has relied for years mainly on fluorescence in situ hybridization. The introduction of other procedures, such as Next-Generation Sequencing has dramatically improved CNAs discovery at genome-wide level. The detection of CNAs by NGS in FFPE material is, nonetheless, a complex issue, which still requires validation studies. Herein, the CNAs detection by a widely used NGS assay (Oncomine Comprehensive Assay plus®, OCA+) were evaluated in 14 FFPE samples mirroring diagnostic daily practice and compared to a whole-genome assay. OCA+, a targeted DNA panel, showed lower CNAs detection sensitivity and equal specificity for gains and losses. According to proprietary software pipeline, OCA+ accurately identified gains characterized by CN ≥ 5,2. No significant threshold maximizing the difference between true and false positive losses was found. Orthogonal FISH tests validated seven CNAs characterized by CN gain ≥ 6 or complete loss. Considering the CNAs growing significance in precision medicine, our findings further prompt towards a robust validation of NGS detection in FFPE materials.
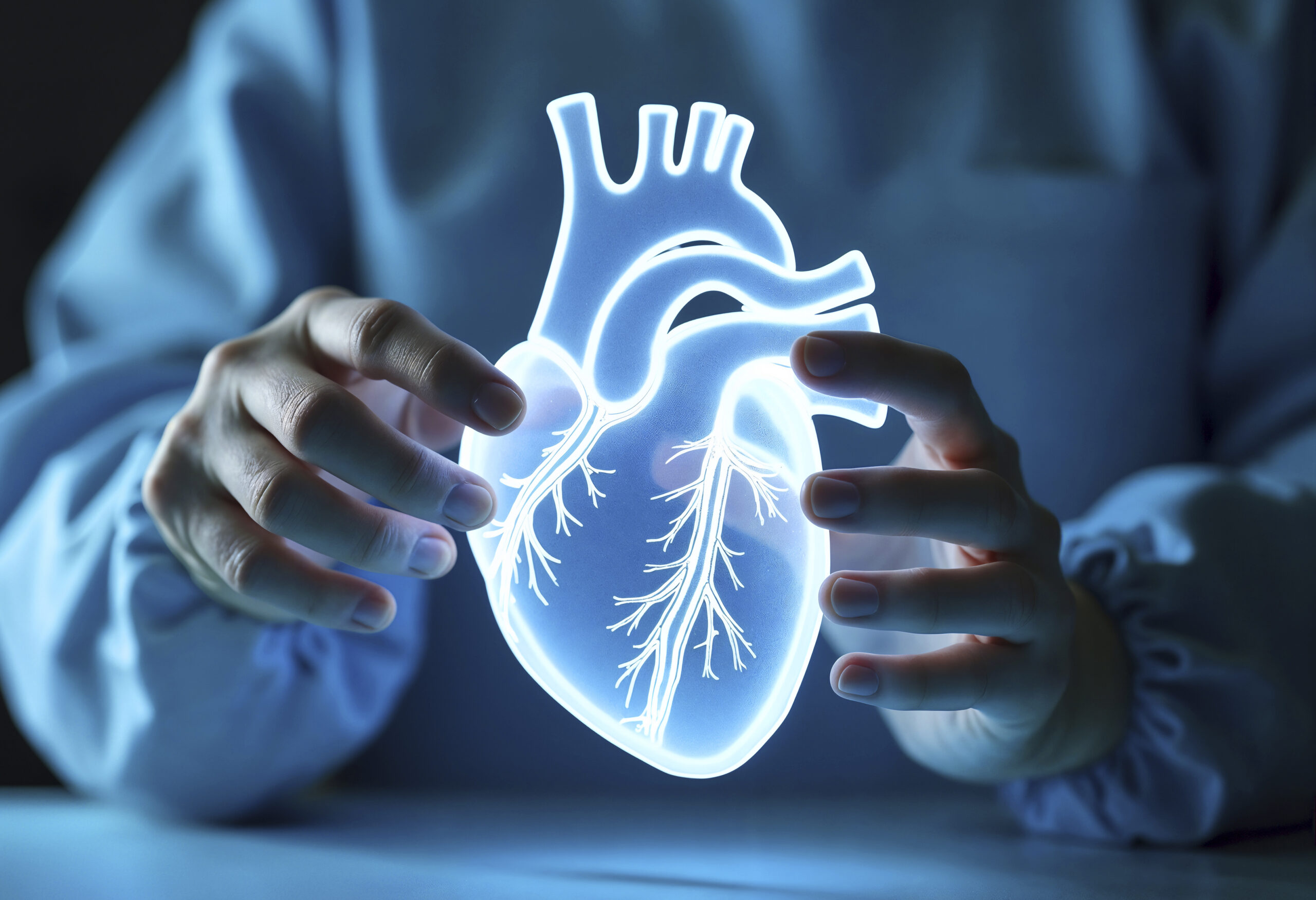
Bimodal ECG-PCG Cardiovascular Disease Detection: a Close Look at Transfer Learning and Data Collection Issues.
Rilevamento bimodale delle malattie cardiovascolari tramite ECG e PCG: un’analisi approfondita sul transfer learning e sulle problematiche di raccolta dati.
Published: 28 NOVEMBER 2024
Bimodal ECG-PCG Cardiovascular Disease Detection: a Close Look at Transfer Learning and Data Collection Issues, A. Calzoni, M. Savardi, A. Signoroni – 3rd AIxIA Workshop on Artificial Intelligence For Healthcare (HC@AIxIA 2024), November 25–28, 2024, Bolzano, Italy
ABSTRACT
Early detection of cardiovascular diseases (CVDs) is crucial for minimizing their adverse impact on patients’ health. Electrocardiograms (ECGs), which capture the heart’s electrical activity, have been widely used to primarily evaluate heart conduction disorders. On the other hand, phonocardiograms (PCGs) recorded during cardiac auscultation, have been less explored, often being overlooked in favor of echocardiograms for detecting mechanical issues such as valvular diseases. However, due to their low cost and non-invasive nature, the analysis of both ECGs and PCGs can be easily integrated into preventive settings. Combining effectively the complementary information from these two modalities could significantly enhance the early detection of CVDs, where Machine Learning (ML) techniques can offer promising and cost-effective solutions. Progress in this area, however, has been limited by the lack of large enough datasets containing both ECG and PCG signals. One objective of this work is to analyze in-depth prior bimodal CVD detection research, identifying key issues to better address data collection and transfer learning limitations. We also propose a different approach to transfer learning for improving heart sound interpretation. Our findings confirm the effectiveness of using both signals to detect abnormal heart conditions. However, we also notice that even a refined transfer learning approach to enhance PCG interpretation is not enough to fully address the issues coming from the lack of bimodal data, indicating the need for further efforts in this direction. Ultimately, our bimodal approach achieved an overall AUROC of 96.4%, exceeding the performance of corresponding ECG-only and PCG-only models by approximately 3% and 10%, respectively. Compared to the other existing approaches, our method demonstrated superior AUROC performance while maintaining a relatively low false-negative rate, which is critical in CVD screening contexts.